Causal Tree Learning For Heterogeneous Treatment Effect Estimation
Given high-dimensional data describing differences in characteristics between individuals, what state-of-the-art ML technique is best suited for estimating differences in causal effects?
In my last post, I discussed heterogeneous treatment effect estimation, a class of causal effect estimation strategies concerned with estimating causal effects which vary given particular values of a set of confounding variables. Specifically, I discussed conditional average treatment effect estimation, or the process of estimating causal effects conditional on certain values of confounding variables. I then discussed matching and sub-classification, which are used to separate out groups of similar observed individuals, some which are treated by an analyzed intervention, and some of which are not. While these groups of individuals can be used to estimate heterogeneous treatment effects, given that their constituents are relatively similar, subdividing observed individuals by large numbers of confounding variables can result in increasingly inaccurate heterogeneous treatment effect estimations, a phenomenon known in the literature as the curse of dimensionality. At the end of that post, I teased a machine learning strategy which could be used to overcome this wretched curse, by optimally selecting a set of confounding variables to individuals by which maximizes the accuracy of a resultant heterogeneous treatment effect estimation. This machine learning strategy, known as Causal Tree Learning is described in depth my my latest post, through text, images, and a few great interactive visualizations. Click below to check it out!
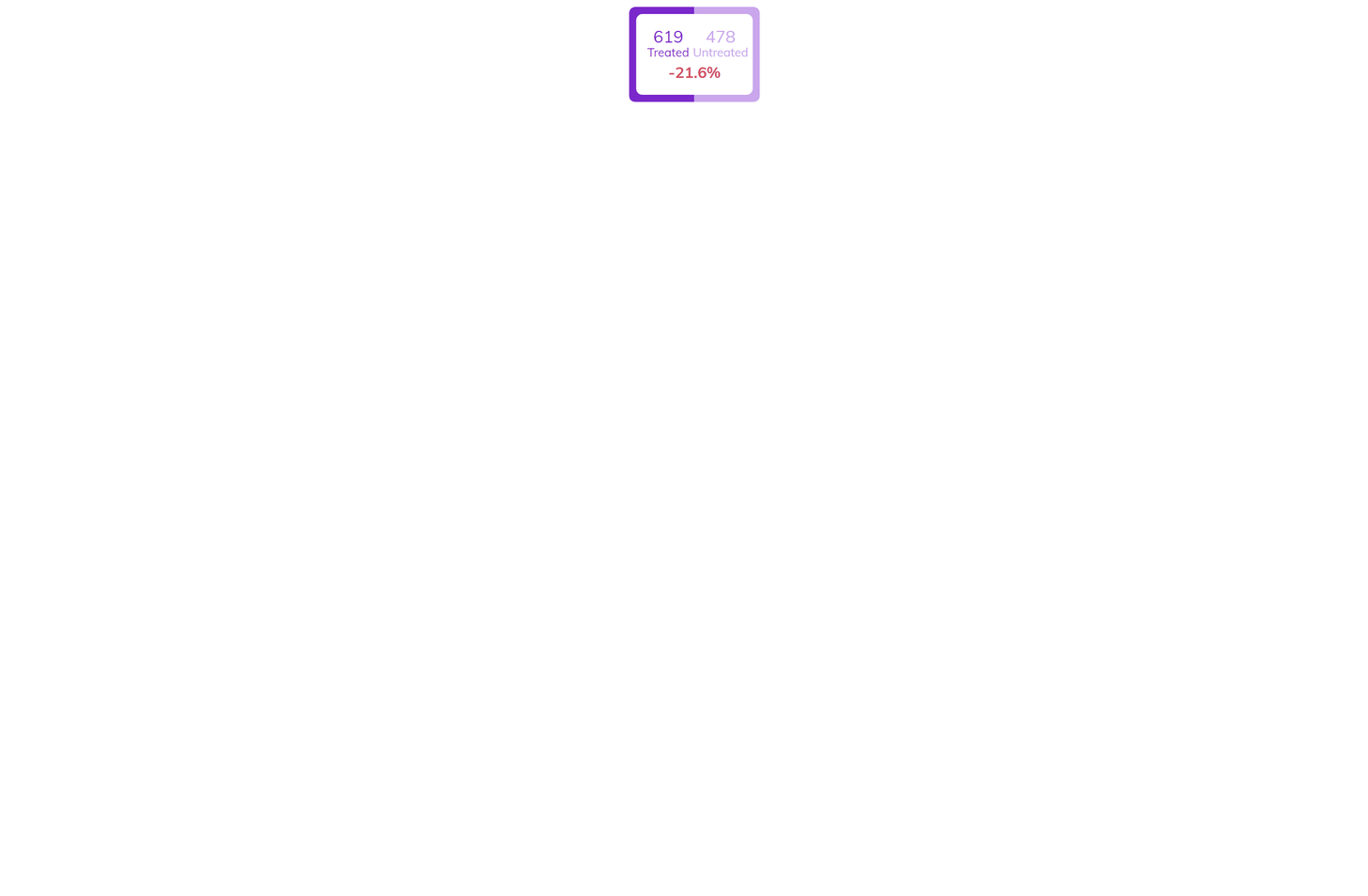
In this post, I present Causal Tree Learning, a machine learning technique developed by the economists Susan Athey and Guido Imbens for automatically estimating heterogeneous treatment effects conditional on a large number of confounding variables. Causal Tree Learning leverages decision trees to identify an optimal strategy to splitting observed individuals into groups in order to estimate heterogeneous treatment effects. Causal Tree Learning has been used to solve causal inference tasks in a variety of business analytics scenarios, particularly for technology-enabled use-cases, such as personalized political messaging campaigns, search advertising optimization, and individualized medical treatment development utilizing large samples of clinical data. The formulation of Causal Tree Learning by Athey and Imbens utilizes two foundational concepts in heterogeneous treatment effects and decision tree learning, which I will describe before explaining how the method can be used for exceptionally accurate causal effect estimation.