Confounding Bias
Why is causal inference so hard? What "adjustments" can we make to observational data in order to make it easier?
In my previous posts, I’ve discussed methodologies for calculating causal effects in scenarios for which we observe an explanatory variable and an outcome variable and wish to quantify a causal relationship. I’ve also discussed how estimates can be biased, as a result of external factors affecting both an explanatory variable and an outcome variable. This bias, called confounding bias, is the main reason why causal inference tasks are so challenging, and for so long, confounding bias has been a significant obstacle to analysts attempts to leverage machine learning techniques for causal inference tasks. In this post, I will formalize our discussion of confounding bias and describe a taxonomy of strategies (front-door and back-door adjustments) for identifying and eliminating it.
Excited to send this one out; once I’ve explained this, I can go more in depth in my discussion of specific estimation and identification methodologies, and can describe how exactly business analysts can use machine learning to understand user behavior from latent observational data.
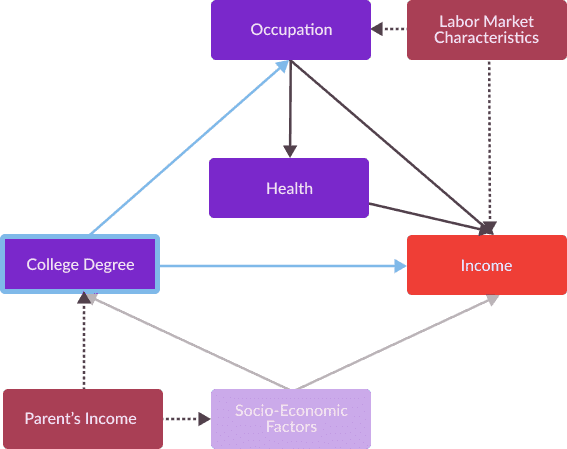