Estimating Average Treatment Effects
How can we estimate average treatment effects and what biases must we be wary of when evaluating our estimation?
In my previous post, I presented a convenient model for describing the counterfactual questions, which ponder the potential outcomes we would see given a change in a particular explanatory variable. In that post I ended the post with an formalization of average treatment effects or the arithmetic mean of all causal effects that a particular explanatory variable may have on individual measurements of an outcome variable. Unfortunately, as a result of the fundamental problem of causal inference, we cannot directly measure average treatment effects. This is because we cannot witness more than one potential outcome, as we cannot set an explanatory variable to more than one value. In my latest post, I discuss the ways in which we can estimate average treatment effects, using data which we can observe. I also describe a set biases which can systematically distort an ATE estimate, and discuss common techniques for eliminating each one of them.
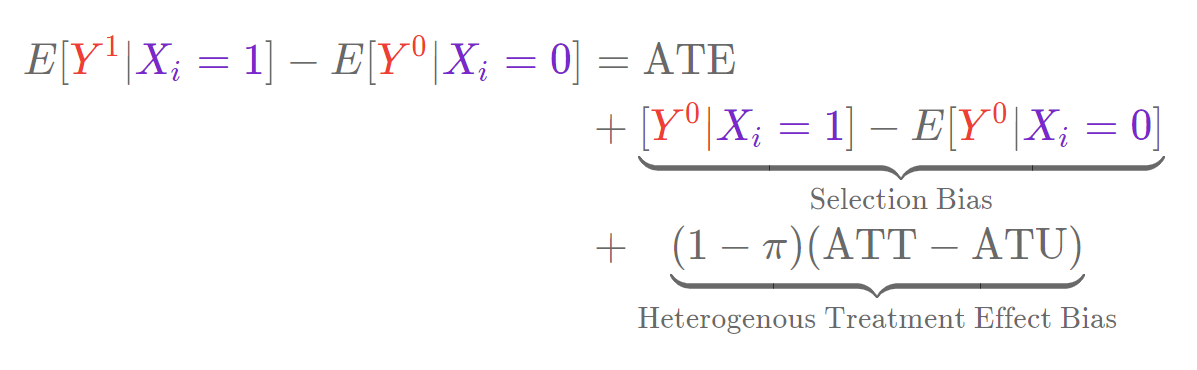
I believe SDO is incorrectly calculated as reader number 7 has 0 for both outcomes, observed and counter factual, with and indicator in 1, so you should add 3 ones and 1 zero and not 4 ones in the selection bias example, am I right? I believe that it would be corrected if you interchange indicators between records 6 and 7.
There are expectation operators missing in the selection bias equation