Potential Outcomes Model
Is there a way we describe the extent of causal relationships, in order to more wholly characterize quantifiable effects?
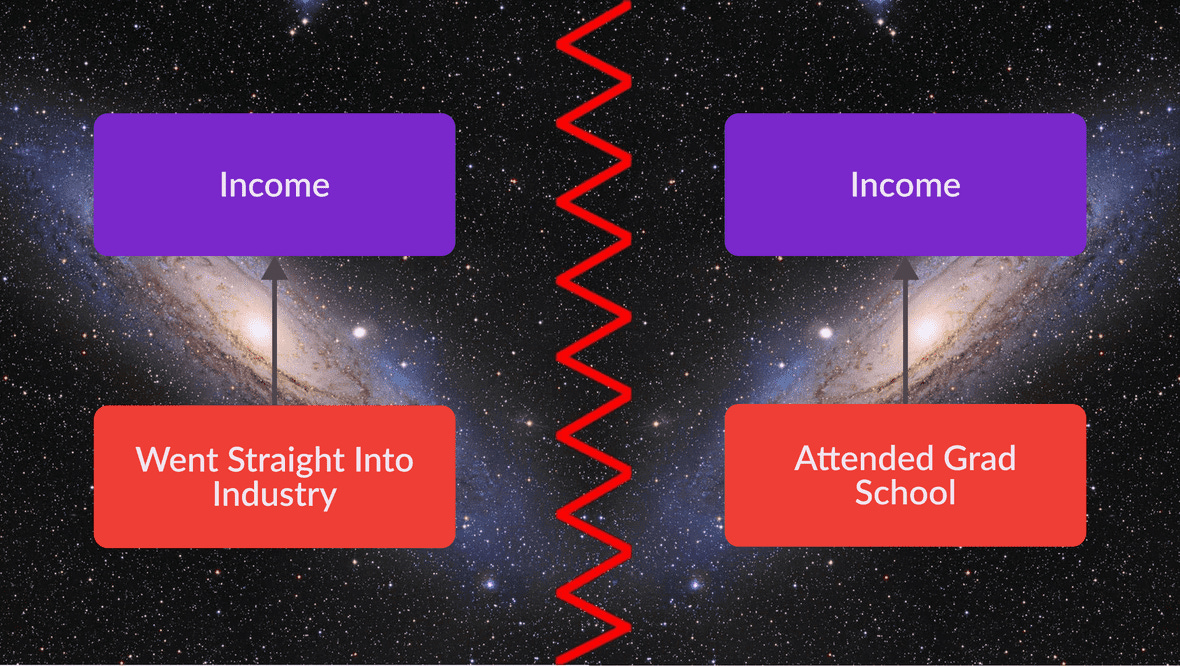
Often times, causal inference analysts are concerned with answering counterfactual questions considering outcomes in a universe we cannot currently observe. The following queries are counterfactual questions:
How much more money would I have made if I attended graduate school?
What is the impact of adding images to my email alerts?
How would moving the ‘Add To Cart’ button effect the purchases made by visitors to an e-commerce site?
If all these questions ponder a hypothetical universe which cannot be measured, what techniques can we use to robustly answer them? In my latest post, I discuss the potential outcomes model, a formalization of counterfactual questions which empowers analysts to generate hypotheses quantifying causal effects. I also discuss average treatment effects a way of characterizing the average extent of an effect on many individuals in a sample population.
Is it “affect” or “effect”?