Propensity Score Matching
How do we "control" for a large number of confounding variables when analyzing causal effects in an observational setting, and have very little control over which individuals receive which treatment?
There is a new post on my blog on causal inference techniques for business analytics! This post is slightly more spruced up than my previous ones! It contains an in-depth explanation of a causal inference application to digital marketing and an interactive visualization meant to illustrate a mathematical concept. Be sure to check it out!
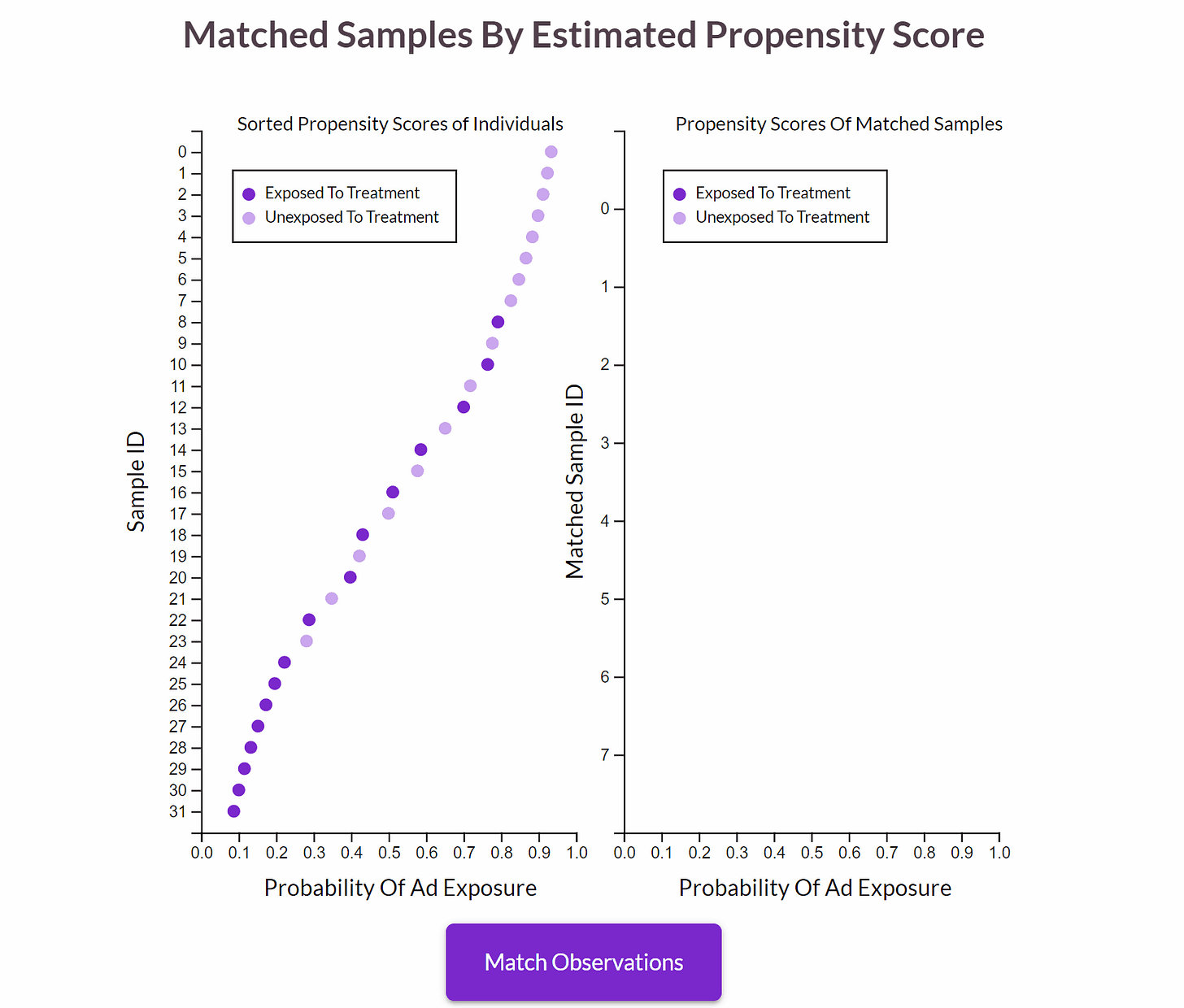
In my previous post, I presented a rigorous definition for confounding bias as well as a general taxonomy comprised of two sets of strategies, back-door and front-door adjustments, for eliminating it. In my discussion of back-door adjustment strategies I briefly mentioned propensity score matching a useful technique for reducing a set of confounding variables to a single propensity score in order to effectively control for confounding bias. Propensity score matching is a quite powerful, rather straightforward backdoor adjustment technique typically used in the presence of selection bias or bias arising from differences between those who are selected to receive treatment and those who are not. Propensity score matching can be used in marketing to estimate a “lift” in revenue due to a particular digital advertising campaign, in medicine to identify the precise effect that smoking has on a variety of health outcomes, and in economics to measure the benefits of socioeconomic support programs in the US. In this blog post and the next, I will present a detailed set of steps for leveraging propensity score matching in order to effectively eliminate confounding bias from a large set of variables.