Propensity Score Matching: What Can Go Wrong?
What are some of the challenges an analyst must be wary of when using propensity score matching for causal inference tasks?
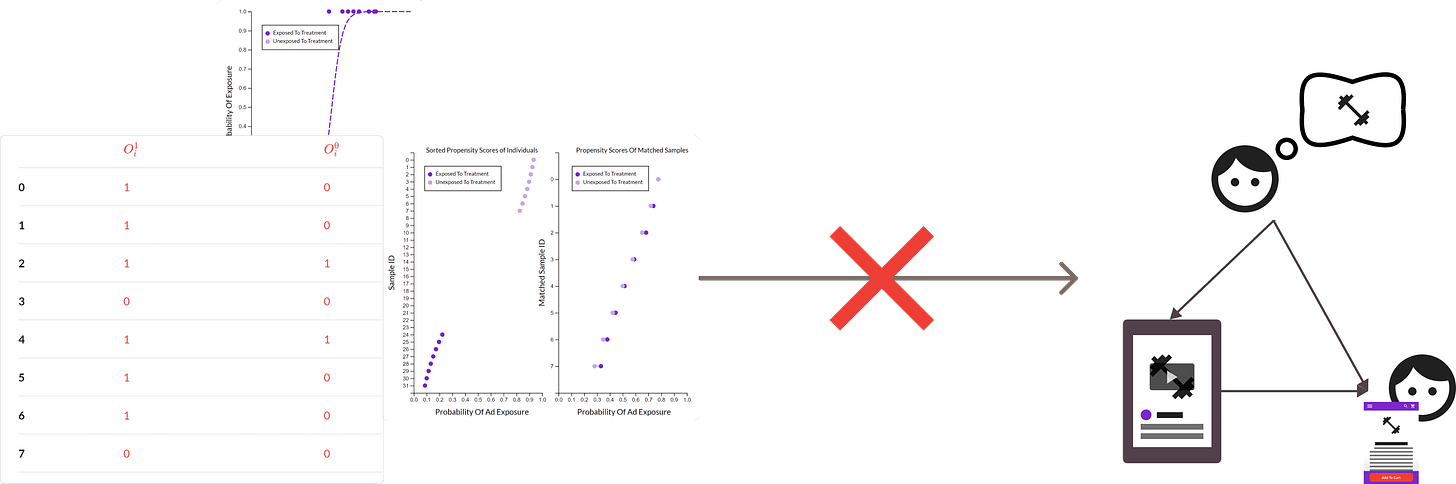
There is a new post on my blog about causal inference for business analytics! Click the button below to check it out! (There’s another interactive visualization!)
This post extends the description of propensity score modelling from my previous post. In that post, I described a scenario in which a marketer may struggle to identify the causal effect of a particular campaign, and discussed a rigorous causal inference technique (propensity score matching) built to solve this problem. In this post, I describe a set of challenges an analyst should be wary of when attempting to estimate a particular causal effect with propensity score matching and present a variety of techniques an analyst can use to ensure their estimate is correct. These challenges, commonly referred to in causal inference literature as, threats to external validity, adversely affect the accuracy of an estimated causal effect, diminishing the utility of propensity score matching technique. Minimizing these threats, using techniques from fields such as translational medicine and machine learning can be a crucial step to guarantee the efficacy of a causal inference estimate. This post should not only provide you with a toolbox for identifying threats to external validity in propensity score matching, but will also give you a framework with which you can consider these threats for every causal inference technique you may encounter. Please check it out if you’re interested in using causal inference for making data-driven decisions — the right way!
Hi this post's content is cut off
Hi there, I think there's something wrong with the UI, the post is not showing.